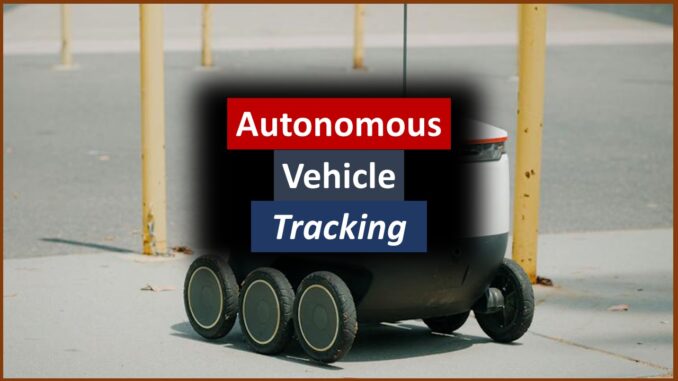
While innovation has been quite apparent in most areas, automotive is where it seems most pervasive. Man has always valued efficient and fast movement as a source of convenience and enjoyment. Of course, many people will talk of going for a drive to clear their head if it’s not to attend to an important matter.
One of the most promising innovations is autonomous cars, which promise to remove errors in your driving. Think of how many accidents self-driving cars will avoid.
But we’re not there yet – just yet! There are a couple of loose ends to take care of. The challenges!
For example, there are challenges around fail-safe oversight, risk assessment, and navigation. Vehicle tracking systems have the lion’s share in making sure the vision of autonomous driving is realised.
The fusion of multifaceted sensor data from tracking solutions with autonomous driving software unlocks innovative new capabilities that accelerate self-driving deployment at scale.
Precise Positioning for Navigation
At the most fundamental level, autonomous vehicles leverage a range of sensors for navigation like cameras and LIDAR. However, radar and sensor data alone does not establish absolute precise positioning – a limitation that can lead to cumulative errors. So, what’s the solution? GPS like PAJ (see their website).
Here GPS tracking fills a critical role in providing regular accurate 3D location fixes to calibrate onboard sensors and self-driving software. They are the perfect eyes that help the vehicles see better and make better on-road decisions.
Having GPS tracking as an extra source of location data makes self-driving car systems more reliable and tough. Most of us have been wary about hacking with autonomous driving.
What happens is that the GPS will give regular location updates from outside the car that combine with the data from the car’s own sensors. This means a hacker would have to trick both systems in the same way to fool the self-driving car about where it is.
Well, doing that consistently at the same time is hard. So checking that the outside GPS data lines up with the sensors inside the car helps to double-check that everything is working right. This consistency between the outside and inside information makes sure the self-driving system knows the right location and is safe for riding in.
The GPS location data also gets plugged into the self-driving car’s sensor fusion engine. This is the smart system inside the car that combines all the different data from sensors that track things like speed, traffic, and obstacles from different angles.
By adding the GPS data into this sensor fusion mix, it allows the self-driving software to make good choices about changing direction or slowing down as needed to reach the destination safely. It takes input from all the devices at once to navigate as the surroundings change.
Having the GPS as an extra check provides very dependable navigation for riders trip after trip. Without blending the tracking data from both outside GPS and built-in sensors, just relying on cameras for self-driving vision could lead to dangerous failures while moving at high speeds.
Fleet Data for Proactive Risk Assessment
In addition to aiding real-time autonomous navigation, data from vehicle tracking solutions applied across entire fleets provides inputs to accelerate software learning. By analysing telemetry data from thousands of autonomous vehicle test runs, AI algorithms gain the necessary digital experience faster to understand normal vs anomalous sensor readings.
What constitutes risky conditions for human drivers like heavy rain or congested highways appear across expansive fleet data for algorithms to incorporate rather than needing to encounter all scenarios firsthand physically.
Disengagement event trackers identify cases where vehicles failed and required human intervention so correlation models can unravel complex combinations of environmental, sensor, and driving factors involved.
Researchers also intentionally expose test vehicles to challenging simulated situations while monitoring reactions via tracking tools to efficiently train systems in a no-risk virtual setting before real-world deployment.
Such fleet-wide data holds tremendous potential for building predictive models that identify signals correlated with increased accident likelihood for advanced early warnings. For example, specific weather data configurations from tracks could act as risk-scoring module inputs to recommend reconsidering trips predicted to be too perilous.
Remote Monitoring for Fail-Safe Oversight
While the end vision is fully autonomous functionality without human oversight needed, the transitionary stages towards full maturity require remote monitoring. This is where GPS tracking brings indispensable transparency.
Detailed real-time telemetry across crucial metrics gets relayed from vehicles to centralised oversight hubs. This allows specialised controllers to ensure proper system health – monitoring everything from compute loads to sensor outputs – and step in promptly if inconsistencies arise.
With holistic visibility into all critical autonomous vehicle parameters throughout trips, remote oversight teams can pinpoint cases of systems misreading environments and pause affected routes for software tweaks before incidents.
Such human-in-the-loop monitoring is crucial for building public confidence in autonomous technology by preventing problems through rapid corrections while technology continuously improves.
Additionally, the capacity for remote intervention via capabilities like fleet-wide speed limits or itinerary adjustments provides emergency safeguards to suspend entire systems instantly if faced with unprecedented situations on the road.
Such fail-safes enabled by tracking offer peace of mind during the ongoing maturation toward full autonomous functionality. Remote analytics on the effectiveness of such overrides provides added data to improve algorithms.
Optimising Autonomous and Manual Fleets
Importantly, companies will continue relying on mixed fleets of autonomous and manually driven vehicles for years with hybrid transition strategies. Here universal tracking solutions that consolidate insights from both autonomous telemetry data and human-operated vehicles provide indispensable unified visibility.
Fleet managers gain holistic operational command centres with the capacity to seamlessly monitor autonomous vehicle progress, and apply remote interventions if needed while maintaining safety and routing optimizations across traditional fleet vehicles.
Instead of independent data silos, hybrid management leveraging vehicle trackers enables seamless oversight driving efficiencies as the balance shifts towards more fully autonomous fleets over time.
With innovators like PAJ GPS leading the charge around implementing next-generation GPS, IoT, and predictive analytics tailored to boost autonomous technology outcomes, the road ahead looks promising. The integration of these multifaceted tracking capabilities with autonomous driving software poises this growing new mobility shift for inevitable success.